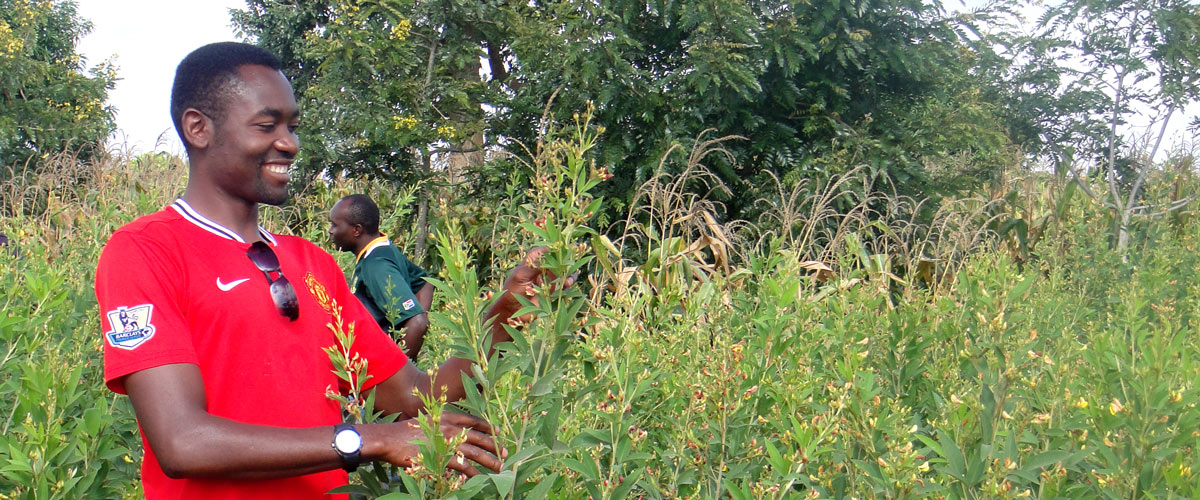
Relearning Research Methods
By Ric Coe, Director and Specialist in Research Methods, Statistics for Sustainable Development
Generations of agricultural scientists have been taught the same research methods. While novel questions sometimes require novel methods, the basic tools have been established a long time. Take field experiments. The principles of good (valid, efficient) experiments were written by Fisher nearly 100 years ago1 and a large part of what we know about the science of agriculture was discovered using these methods. They work!
It’s hardly surprising, then, that many agricultural scientists struggle to reconcile their training with the adaptations that new ways of working with farmers demand. Such doesn’t mean that change isn’t necessary or valuable. Our research methods are only useful to the degree they meet the objectives of a given study. As the role of farmers in research expands and objectives evolve, so should our willingness as researchers to relearn research methods.
On-farm experimentation has been part of agricultural development with smallholders for a long time.2 The emergence of movements such as farmer research networks (FRNs), however, brings new dimensions to the practice. These networks blend everyday learning, research and science, and social exchange and networking. Farmers and researchers collaborate to explore the potential of farmer-centered research to transform food systems, improve livelihoods, build climate resilience, and even reimagine research.
In my own work with FRNs in East Africa, it’s common to hear from researchers that participatory on-farm experiments aren’t effective. They claim that there’s often a lot of variation in what farmers do. Sometimes the treatments they want to compare aren’t “right,” random allocation of treatments may be difficult, and so on. In other words, researchers often feel that farmers’ experiments fail to follow well-known principles for good experimentation and we need to do something about that.
One response is to teach farmers more about the principles of experimentation. Some years ago we put together this simple list of principles and explanations, which some FRNs have found useful.
But there’s another response that’s more important. Established principles for experimental design are based on a frame of reference within which sit the objectives of the experiment. The researcher’s assumption is that there is a true, fixed “treatment effect” and the aim of the experiment is to estimate that as precisely as possible. If that really is the aim, then an experiment that follows Fisher’s principles is what you need. But that’s usually not the context and aim of experiments done by farmers within a FRN.
Consider this example from Malawi: Farmers are used to intercropping maize and a legume such as groundnut or pigeonpea. The idea arose that it might be preferable to mix two different legumes such as groundnut and pigeonpea in the intercrop. The FRN set out to do experiments to compare the use of single and doubled-up legumes. Farmers know there isn’t a single effect of the difference between these practices; that it will probably depend on several other factors, such as soil type and its health, whether it’s a drier or wetter season, whether crops were planted early or late, perhaps the state of weeds in the field.
Here, the objectives become more complex and include gaining insights into the conditions when such doubled-up legumes are a good idea or not. In this case, the variation between farms, rather than being a source of “noise” to be eliminated, can be a source of information that can reveal or suggest reasons for some of the complexity. The old principle of “controlling variation” no longer looks so obviously sensible.
Another common complaint about farmers’ experiments is that treatments are not always identical across all farms. That can happen for several different reasons. For example, an experiment is set up to compare a new practice with current practice. That sounds easy. But what if the “current practice” is different on different farms?
The classical researcher’s approach is to choose a single standard with which to compare the new practice—sometimes the most common practice, sometimes the recommended practice (even if that isn’t what farmers use). However, if we think of the experiment as providing information for the participating farmers, comparing the new practice with their own individual current practice makes sense to farmers because it is most relevant to their own decision-making. The collective information from the trial then includes data on the percentage of farmers who found the new practice better than their current one, perhaps allowing identification of who those farmers are and in what ways it was “better.”
The common pattern here is that the aims of participatory on-farm experiments are likely to differ from the aims experiment researchers would do on their own. That means the appropriate design and methods also need to differ, with the standard tools perhaps not being useful and the principles on which they are based requiring update.
The implication is that researchers working in these collaborative networks may need to relearn research methods. Rather than assuming that “farmers can’t do experiments” or that they need to learn “our“ methods, we must take a careful look at the aims of research and the principles and procedures that will meet those aims.
Researchers often need to think carefully about why they do things the way they do and how that can be aligned with the reality of research by an FRN. This relearning is not easy. Despite research being about innovation and discovery, it can be a conservative process with inertia imposed by teaching curricula, standard practices in research organizations, or the expectations of science publishers. If collaborative networks like FRNs are to have a fruitful relationship with science, then researchers need to adapt their concepts of what good research looks like.
Notes:
- Fisher, R.A. Statistical Methods for Research Workers (Edinburg: Oliver and Boyd, 1925)
- Ashby, J.A. Evaluating Technology with Farmers: A Handbook (Cali, Colombia: CIAT, 1990)
Date:
10/24/2021